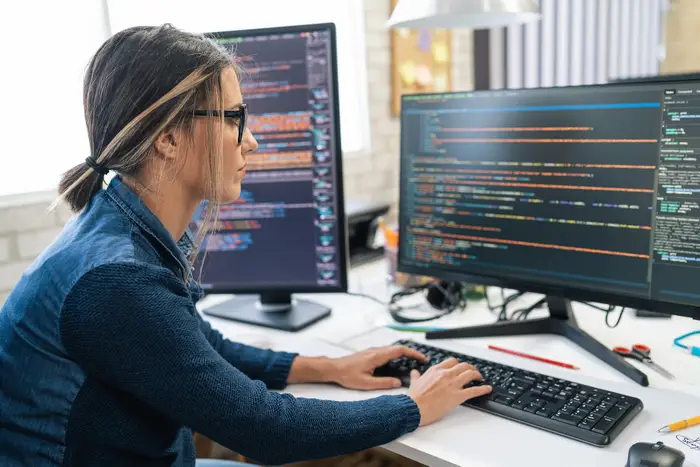
Unprecedented Bidding War Erupts Over Anysphere, Creator of Popular AI Coding Assistant Cursor
Technology News
Zaker Adham
09 November 2024
07 August 2024
|
Zaker Adham
Summary
Summary
In today's world, the explosion of data and user-generated content across various media platforms—from social networks to news sites, e-commerce reviews, and niche forums—has made it crucial to accurately interpret the emotions conveyed in these messages. This capability is increasingly important in fields such as social science, politics, marketing, business, and economics.
Recent progress in "sentiment analysis" has led to the creation of more advanced models that can extract and interpret emotional nuances in textual data. One notable model is the BERT-ABiLSTM, which stands for Bidirectional Encoder Representations from Transformers, Attention Bidirectional Long Short-Term Memory.
A study published in the International Journal of Information and Communication Technology explores how this large-scale pre-trained algorithm can be utilized for sentiment analysis. However, as Zhubin Luo from the Hunan University of Humanities, Science and Technology in China notes, the ABiLSTM component has some limitations, as it focuses on global features and may miss subtle nuances.
BERT, according to Luo, can learn language representations from extensive text corpora. The ABiLSTM, a type of recurrent neural network, processes text sequences. Luo has enhanced the system by incorporating TextCNN (Text Convolutional Neural Network) to create BERT-CNN-ABiLSTM, a more sophisticated version of the model. This bidirectional approach allows the model to understand context from both past-to-future and future-to-past segments of text, which is crucial for capturing long-term dependencies. The attention mechanism within ABiLSTM further refines this by enabling the model to focus on the most relevant parts of the text, thereby improving sentiment analysis accuracy.
The TextCNN component uses convolutional kernels of various sizes to detect different granularities of features within the text. This enables the model to capture subtle local patterns that simpler models might miss, providing a more detailed analysis of textual content.
Luo's improvements are particularly significant for applications requiring detailed text classification and recognition, such as sentiment analysis on social media, evaluating customer feedback on e-commerce platforms, or enhancing "intelligent" online question-and-answer systems.
Technology News
Zaker Adham
09 November 2024
Technology News
Zaker Adham
09 November 2024
Technology News
Zaker Adham
09 November 2024
Technology News
Zaker Adham
07 November 2024