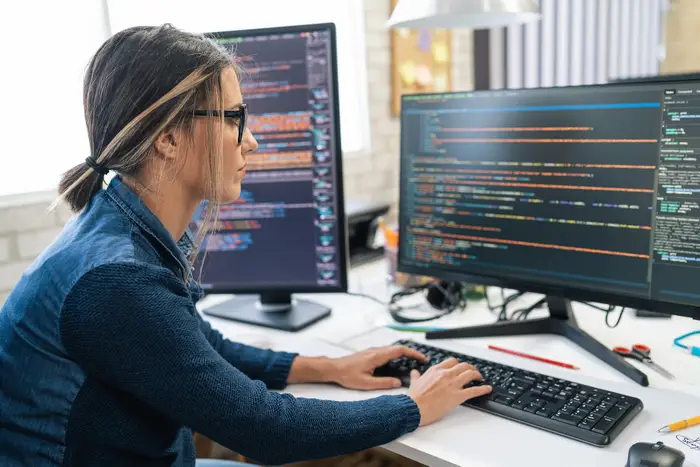
Unprecedented Bidding War Erupts Over Anysphere, Creator of Popular AI Coding Assistant Cursor
Technology News
Zaker Adham
09 November 2024
03 November 2024
|
Zaker Adham
Summary
Summary
This week, MIT researchers introduced an innovative model for teaching robots. Departing from traditional methods that rely on narrowly focused data, the team’s new approach draws inspiration from large language models (LLMs), utilizing vast datasets to enhance adaptability. This technique could allow robots to tackle more complex tasks by mimicking the extensive data training used in language models like GPT-4.
The existing technique of imitation learning, where robots learn by observing tasks, often struggles with environmental variations, such as changes in lighting, settings, or unexpected obstacles. These factors can cause imitation learning to falter since robots trained with limited data may lack the flexibility to adapt.
To address this, the MIT team looked to language models as a guide. “In the language domain, the data are all just sentences,” explained Lirui Wang, the lead author of the study. “In robotics, given all the heterogeneity in the data, if you want to pretrain in a similar manner, we need a different architecture.”
To tackle the need for a versatile architecture, the team developed a system called Heterogeneous Pretrained Transformers (HPT). This new design gathers data from a wide range of sensors and settings, consolidating the information to train robots more effectively. Using transformers, HPT integrates various types of data, enabling better performance across different robotic tasks. The larger the dataset and the model, the more capable the robot becomes in executing complex operations.
This model allows users to specify the robot’s design, configuration, and task, with the system adapting accordingly. The goal, as described by CMU associate professor David Held, is “a universal robot brain that you could download and use for your robot without any training at all.” While the technology is still in its early stages, Held expressed optimism about the potential breakthroughs this model could bring to robotic training, similar to advancements seen with large language models.
This research received funding from the Toyota Research Institute (TRI), which has been actively supporting innovations in robotic learning. Last year, TRI demonstrated an overnight robot training method and recently partnered with Boston Dynamics to integrate its research into advanced robotic hardware.
This new collaboration signifies an important step in scaling up robotic training capabilities. By adopting a data-rich approach, researchers hope to develop adaptable, versatile robots capable of performing a wide array of tasks across different environments.
Technology News
Zaker Adham
09 November 2024
Technology News
Zaker Adham
09 November 2024
Technology News
Zaker Adham
09 November 2024
Technology News
Zaker Adham
07 November 2024