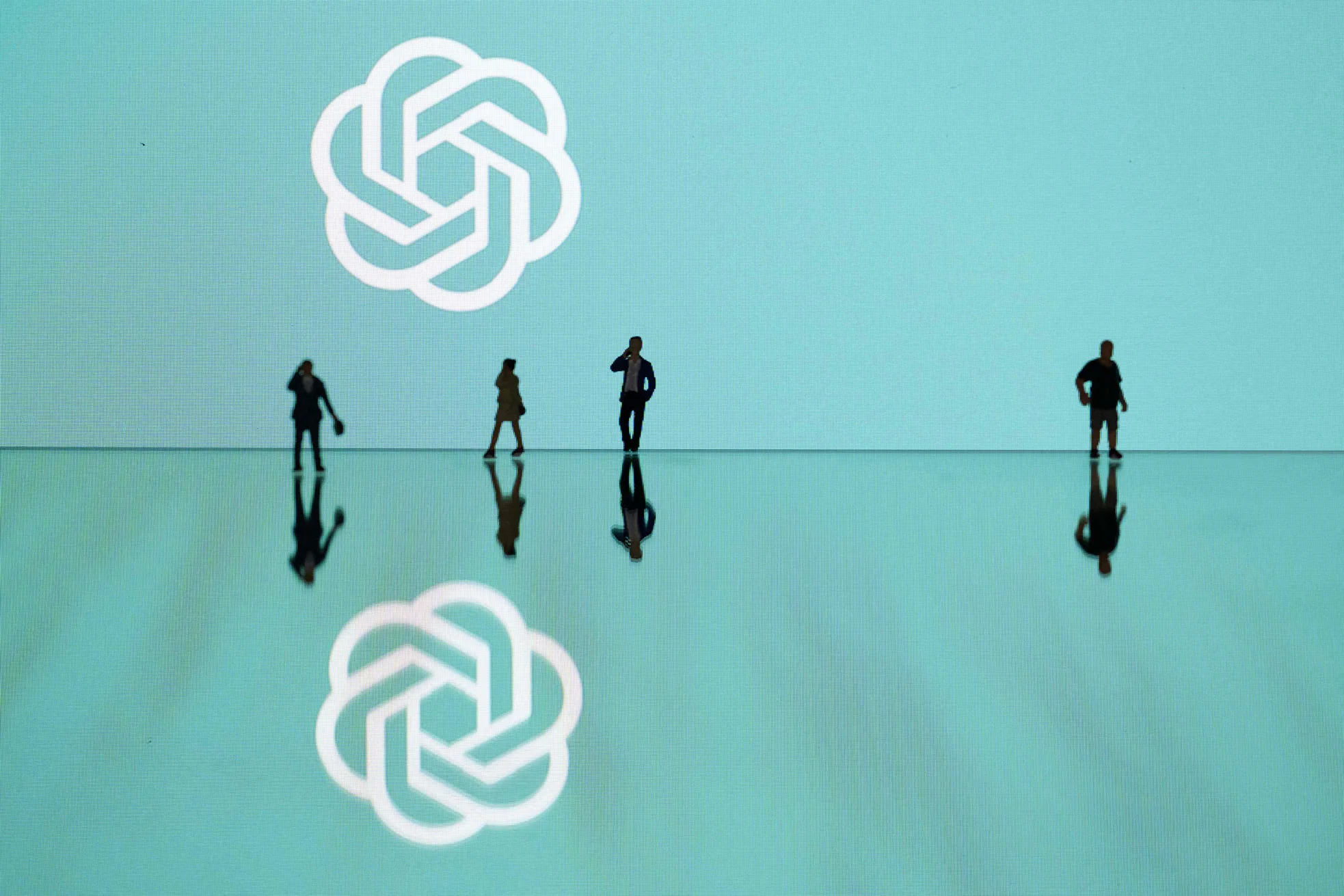
ChatGPT Sends Millions to Verified Election News, Blocks 250,000 Deepfake Attempts
AI
Zaker Adham
09 November 2024
06 August 2024
|
Zaker Adham
Summary
Summary
With the rise of generative AI tools like ChatGPT, distinguishing between genuine and fake scientific articles has become increasingly challenging. Ahmed Abdeen Hamed, a visiting research fellow at Binghamton University's Thomas J. Watson College of Engineering and Applied Science, has developed a machine-learning algorithm named xFakeSci. This tool can identify up to 94% of fraudulent papers, significantly outperforming traditional data-mining techniques.
Hamed, whose primary research is in biomedical informatics, emphasizes the importance of verifying the authenticity of medical publications, clinical trials, and online resources. During the global pandemic, the spread of false biomedical research became a significant concern.
In a study published in Scientific Reports, Hamed and Xindong Wu, a professor at Hefei University of Technology in China, generated 50 fake articles on Alzheimer's, cancer, and depression. They compared these to an equal number of genuine articles on the same topics. By analyzing the frequency and connections of bigrams (pairs of words that often appear together), xFakeSci was able to detect patterns unique to fake articles.
Hamed explains that AI-generated papers tend to use fewer bigrams, but these are highly interconnected, unlike genuine research papers. This difference in writing style is due to the distinct goals of human researchers and AI systems.
Mohammad T. Khasawneh, Distinguished Professor and Chair of the Department of Systems Science and Industrial Engineering, praised Hamed's innovative work. He highlighted the relevance of this research in an era where deepfakes are a growing concern.
Hamed plans to expand xFakeSci's capabilities to cover a broader range of topics beyond medicine, including engineering and the humanities. He acknowledges that as AI technology advances, detecting fake content will become more challenging, necessitating continuous improvements to detection algorithms.
AI
Zaker Adham
09 November 2024
AI
Zaker Adham
09 November 2024
AI
Zaker Adham
07 November 2024
AI
Zaker Adham
06 November 2024